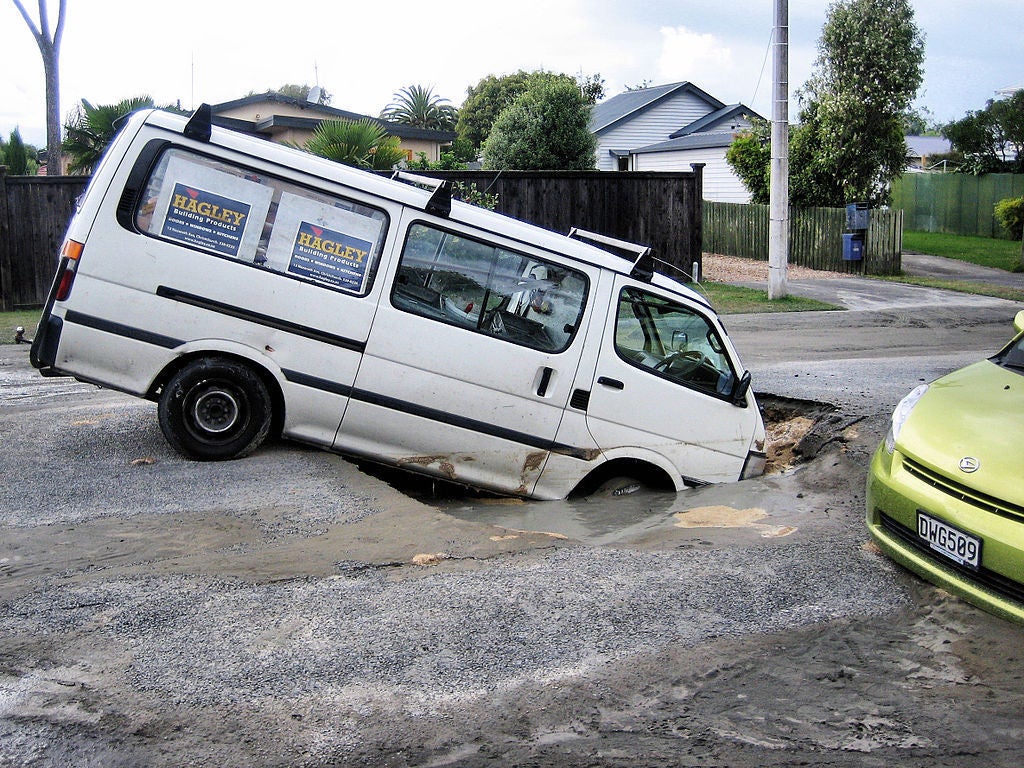
AUSTIN, Texas — Our homes and offices are only as solid as the ground beneath them. When that solid ground turns to liquid — as sometimes happens during earthquakes — it can topple buildings and bridges. This phenomenon is known as liquefaction, and it was a major feature of the 2011 earthquake in Christchurch, New Zealand, a magnitude 6.3 quake that killed 185 people and destroyed thousands of homes.
An upside of the Christchurch quake was that it was one of the most well-documented in history. Because New Zealand is seismically active, the city had numerous sensors for monitoring earthquakes. Post-event reconnaissance provided a wealth of additional data on how the soil responded across the city.
Two researchers from The University of Texas at Austin developed a machine learning model that predicted the amount of lateral movement that occurred when the Christchurch earthquake caused soil to lose its strength and shift relative to its surroundings.
The results were published online in Earthquake Spectra in April 2021.
“It’s one of the first machine learning studies in our area of geotechnical engineering,” said postdoctoral researcher Maria Giovanna Durante, a Marie Sklodowska Curie fellow previously at UT Austin. “It’s an enormous amount of data for our field. If we have thousands of data points, maybe we can find a trend.”
Durante and Ellen Rathje, the Janet S. Cockrell Centennial Chair in Engineering at UT Austin and the principal investigator for the National Science Foundation-funded DesignSafe cyberinfrastructure, first used a Random Forest approach with a binary classification to forecast whether lateral spreading movements occurred at a specific location. They then applied a multiclass classification approach to predict the amount of displacement, from none to more than 1 meter.
“It was important to select specific input features that go with the phenomenon we study,” Durante said. “We’re not using the model as a black box — we’re trying to integrate our scientific knowledge as much as possible.”
Durante and Rathje trained the model using data related to the peak ground shaking experienced (a trigger for liquefaction), the depth of the water table, the topographic slope, and other factors. In total, more than 7,000 data points from a small area of the city were used for training data — a great improvement, as previous geotechnical machine learning studies had used only 200 data points.
They tested their model citywide on 2.5 million sites around the epicenter of the earthquake to determine the displacement. Their model predicted whether liquefaction occurred with 80% accuracy; it was 70% accurate at determining the amount of displacement.
The researchers used the Frontera supercomputer at the Texas Advanced Computing Center (TACC), one of the world’s fastest, to train and test the model. TACC is a key partner on the DesignSafe project, providing computing resources, software and storage to the natural hazards engineering community.
Access to Frontera provided Durante and Rathje machine learning capabilities on a scale previously unavailable to the field. Deriving the final machine learning model required testing 2,400 possible models.
“It would have taken years to do this research anywhere else,” Durante said. “If you want to run a parametric study or do a comprehensive analysis, you need to have computational power.”
"type" - Google News
June 24, 2021 at 12:33AM
https://ift.tt/3zPDVdz
New Type of Machine Learning Aids Earthquake Risk Prediction - UT News - UT News | The University of Texas at Austin
"type" - Google News
https://ift.tt/2WhN8Zg
https://ift.tt/2YrjQdq
Bagikan Berita Ini
0 Response to "New Type of Machine Learning Aids Earthquake Risk Prediction - UT News - UT News | The University of Texas at Austin"
Post a Comment